What is Sentiment Analysis? Tools and Uses
Businesses are drowning in data while starving for insights.
Every day, your customers leave digital breadcrumbs about how they feel about your brand—in comments, reviews, emails, and support tickets.
They tell you exactly what they think—what makes them loyal or pushes them away.
But can you hear them? Can you make sense of thousands of voices speaking at once?
This is where sentiment analysis enters the game. It's not just another tech buzzword—it's the difference between flying blind and having radar that detects customer emotions before they impact your bottom line.
In this article, I'll explain exactly what sentiment analysis is, the tools revolutionising how businesses use it, and the specific ways you can deploy it to create an unfair advantage in your market.
Whether you're looking to reduce churn, improve product-market fit, or understand what your customers want (not what they say), sentiment analysis might be the missing piece in your business intelligence toolkit.
- Sentiment analysis identifies customer emotions, helping businesses respond proactively to improve customer loyalty and brand reputation.
- AI tools like NLP and machine learning facilitate real-time sentiment detection across industries, from marketing to finance.
- Ethical considerations, such as privacy and bias, are crucial for responsible implementation of sentiment analysis technologies.
- Advanced techniques like hybrid models and aspect-based analysis provide deeper insights, enhancing decision-making and understanding customer perspectives.
Core Concepts and Applications

Here's the deal—if you're running a business and you're not paying attention to what people feel (not just what they say), you're flying blind.
Sentiment analysis is the tech that does the emotional heavy lifting. It tells you if people love you, hate you, or don't care. And it does it fast. We're talking about AI that scans text—social posts, reviews, emails, forum posts—and figures out what is being said and how it's being said. Positive, negative, sarcastic, emotional—whatever the vibe, it picks it up.
Here's why this matters:
- It can detect customer happiness before your sales dip.
- It catches brand damage before you even notice it.
- It reveals buying intent before the person hits your landing page.
We're not just talking “thumbs up” or “thumbs down”. We're talking deep emotion mapping—joy, anger, frustration, surprise. It even picks up sarcasm (basically the internet's love language).
Where It's Being Used (Hint: Everywhere That Matters)
You'll find sentiment analysis in every high-leverage industry. Why? Because emotions drive actions, and actions drive revenue. Here are just a few places where it's already making money:
- Marketing: Build campaigns that resonate. Kill what doesn't.
- Customer Service: Know which customers are ticking time bombs—and save them.
- Brand Management: Measure how people feel about your brand in real time.
- Finance: Hedge funds use this to predict market moves based on public mood. Crazy, right?
- Public Health: Spot flu outbreaks or mental health trends can be analysed by analysing what people post.
- Politics: Understand voter sentiment way before the polls show it.
- Defence: Track sentiment in unstable regions to prevent escalations.
This isn't sci-fi. It's happening right now.
And with real-time sentiment analysis, it's getting even wilder. Brands watch social media mentions as they happen, reacting within minutes—not days. It's the difference between a PR win and a PR disaster.
Oh, and it moderates online communities, too—catching toxicity before it spreads.
The Engine Behind the Magic
Let's break this down. Sentiment analysis is a Frankenstein built from multiple disciplines:
- NLP (Natural Language Processing)
- Machine Learning
- Deep Learning
- Computational Linguistics
- Data Mining
- Even Biometrics (yes, physical cues from text)
It's not just AI nerds—computer science + psychology + behavioural economics. Do you want the best results? You need to understand both the machine and the human.
Ethics: Don't Be Creepy
If you're using this tech, you need to play it straight. Privacy, consent, bias—these aren't just checkboxes. If you mess this up, it will come back to bite you.
The biggest mistake? Feeding biased data into your model and expecting fair outcomes. Garbage in, garbage out.
Solution? Build-in checks. Be transparent. Test your systems regularly. Otherwise, you're scaling a problem instead of a solution.
So, What Does Sentiment Analysis Do?
At its core, it does two main things:
- Subjectivity Detection – Is this statement based on fact or opinion?
- Polarity Detection – Is that opinion positive, negative, or neutral?
But that's just the baseline. Great systems go further:
- Measure how positive or negative something is.
- Classify reviews by type (product, service, entertainment, etc.)
- Detect specific emotions: joy, sadness, anger.
- Spot sarcasm (a marketer's worst enemy).
- Summarise how people feel about your brand, service, or CEO—at scale.
- Track sentiment trends over time.
- Break down sentiment by demographic group.
- Explain why people think the way they do (this is the goldmine).
If you care about what customers think—and should—then sentiment analysis isn't lovely. It's a profit lever.
Because in business, it's not just about being good. It's about being liked, trusted, and remembered.
And can you read emotion at scale?
You win.
- Hardcover Book
- Liu, Bing (Author)
- English (Publication Language)
Key Techniques and Methods
There are several major approaches used to build sentiment analysis systems:
- Lexicon-based – Uses sentiment lexicons containing words/phrases labelled as conveying positive sentiment, negative sentiment, etc.
- Machine learning – Trains statistical machine learning models like logistic regression on labelled sentiment data.
- Deep learning – Leverages neural networks to understand the sentiment in text based on learnt semantics.
- Hybrid – Combines machine learning and deep learning with lexicon-based approaches.
Additionally, here are some other notable techniques:
- Aspect-based – Detects sentiment towards specific attributes of products/services
- Multilingual – Handles multiple languages like English, French, and Chinese
- Multimodal – Incorporates non-textual data like images, audio, video
- Transfer learning – Leverages models pre-trained on huge text entities
Later sections provide more details on these approaches and innovations in sentiment analysis methodology. First, let’s explore the building blocks that enable sentiment analysis.
Foundations of Sentiment Analysis
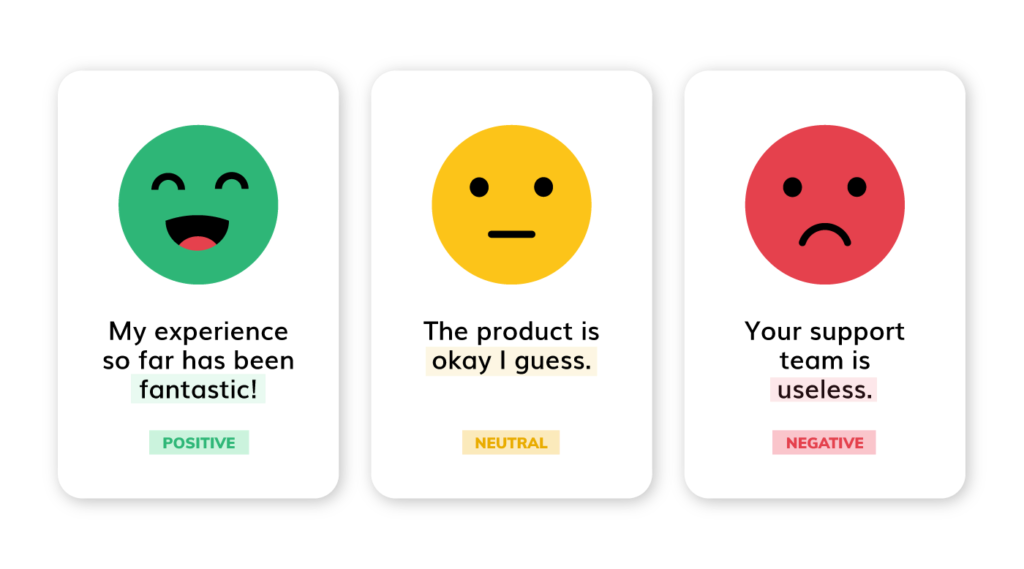
Sentiment analysis has foundations across linguistics, text processing, data science, machine learning, and evaluation metrics. Here, we introduce fundamental concepts that serve as building blocks for sentiment analysis systems.
Linguistic Foundations
- Parts-of-speech – Nouns, verbs, adjectives, adverbs, etc. Adjectives frequently convey sentiment.
- Negation handling – Negation words like “wouldn’t” can flip sentiment orientation.
- Word sense disambiguation – Determining word meaning from context to assess connotative sentiment.
Text Processing
- Tokenisation – Splitting text into words, phrases, symbols, etc.
- Normalisation – Converting text to the typical case, stripping punctuation/whitespace, etc.
- Stopword removal – Removing frequent words like “and” or “the” that rarely convey sentiment.
Feature Engineering
- N-grams – Contiguous word sequences useful for capturing context.
- TF-IDF vectors – Statistics reflect the importance of terms in documenting in a corpus.
- Word embeddings – Encode words/phrases into dense vector representations capturing meaning.
Machine Learning Models
- Logistic regression – Predicts probabilities of class membership.
- Naive Bayes classifier – Probabilistic model based on Bayes Theorem.
- SVM – Finds optimal line/plane to separate classes.
- RNNs – Recurrent neural network architecture well-suited for sequence data.
Evaluation Metrics
- Accuracy – Percentage of correct polarity classifications.
- Precision – Positive precision measures the percentage of identifying true positives.
- Recall – Percentage of actual positive cases correctly classified
- F1 scores – Balance between precision and recall.
These concepts establish the foundation for the sentiment analysis techniques explored next.
- Pozzi, Federico Alberto (Author)
- English (Publication Language)
- 284 Pages – 09/30/2016 (Publication Date) – Morgan Kaufmann (Publisher)
Key Sentiment Analysis Techniques
Now that we’ve covered sentiment analysis's integral tasks and foundations let’s dig deeper into the prevailing techniques that are leveraged today.
Lexicon-Based Approaches
Lexicon-based techniques rely on sentiment lexicons – dictionaries containing words/phrases annotated with polarity scores. For example:
Term | Polarity Score |
wonderful | +5 |
garish | -3 |
Lexicons can assign polarity scores at various linguistic levels:
Document Level
Aggregate word-level polarity scores to determine overall document sentiment. For example:
This hotel had beautiful views, but the tacky decor ruined the experience.
Positive words: {wonderful: +5}
Negative words: {garish: -3; ruined: -2}
- -5 negative points
- +5 positive points
- Net Negativity
Challenges with lexicon methods include handling negation and accounting for shifts in word polarity based on context. Many lexicons also have limited vocabulary coverage.
Machine Learning Approaches
Machine learning classifiers for sentiment analysis are trained on labelled datasets containing text samples annotated with their sentiment. Common models include:
- Logistic regression
- Naive Bayes
- Support Vector Machines (SVMs)
For example, an SVM model might be trained to classify movie reviews as positive or negative based on word n-gram features:
Review Text | Labeled Sentiment |
This film was imaginative and impactful. | Positive |
The plot was dull and predictable. | Negative |
Once trained, these models can classify the sentiment of new unlabeled reviews. Machine learning approaches excel at incorporating features that capture negation, shifts in word sense based on context, etc. However, they rely on quality training data.
Deep Learning Methods
Let's summarise: traditional sentiment analysis is deadweight if you still rely on word counts and keywords.
The new era? Deep learning.
Tools like RNNs (Recurrent Neural Networks) and LSTMs (Long Short-Term Memory Networks) don't just count words—they understand them. These models don't need a ton of data cleaning or handholding. They learn sentiment by actually grasping the meaning behind the language. Not just what's being said—but how it's being said and why.
So now you're not looking at “happy” = positive or “hate” = negative. You're looking at context-driven emotion extraction. And that's the difference between surface-level guesses and insights that help you make decisions.
Transformers: The Big Guns
Then came transformer models like BERT and GPT—and everything changed.
These models look at the entire sentence or paragraph as a whole. That means they don't just understand one word at a time; they understand relationships between words, no matter how far apart they are in the sentence.
Think about understanding sarcasm, mixed emotions, or someone saying the exact opposite of what they mean. That's real context. That's actual value.
And thanks to transfer learning, you don't need to train these beasts from scratch. They come pre-trained on mountains of data. You fine-tune them for your niche—healthcare, finance, e-commerce, whatever—and they outperform everything else.
Here's What Most People Get Wrong
Just because you're using GPT or BERT doesn't mean you're winning. You still need:
- Smart tuning
- Domain expertise
- Understanding the language of your industry
Context matters. “Bullish” in finance ≠ “bullish” in everyday chat. So if your model's not trained with that lens, it'll get it wrong—and you'll act on garbage data.
What's Next? Hybrid Power Plays
Some of the most innovative companies combine deep learning with classic NLP techniques. Think of it like this:
- Deep learning handles the nuance (emotion, context, sarcasm)
- Lexicon-based methods and traditional classifiers catch the rules and patterns
Together, they create hybrid systems that outperform either method on its own. It's not about AI vs human—it's AI with logic.
Also worth noting: more models are being trained using transfer learning. This is like giving your sentiment model a PhD in linguistics before it starts reading your data. It knows the rules of language out of the gate. All you have to do is teach it the dialect of your specific use case.
Industry-Specific Sentiment = Custom Cash Machines
Now let's talk verticals—because generic solutions don't scale.
- Healthcare: Sentiment tools dig into patient feedback, surfacing what's broken in service delivery before it shows up in hospital reviews.
- Finance: Models track investor sentiment and news tone to predict economic shifts and market moves. Yes, people are making trades off this stuff.
- Entertainment: Studios use it to track how audiences feel about characters, plot lines, trailers—you name it.
Bottom line: Sentiment analysis is your edge if your industry has opinions.
Wrap-Up: Use the Right Tools to Hear What People Aren't Saying
The biggest unlock here isn't the tech. It's the insight.
Deep learning + transformers + industry-specific tuning = a level of understanding that turns passive data into actionable direction.
If you know how your audience feels—even when they don't say it directly—you can sell better, serve better, and build faster.
That's the actual ROI.
New Frontiers in Methodology
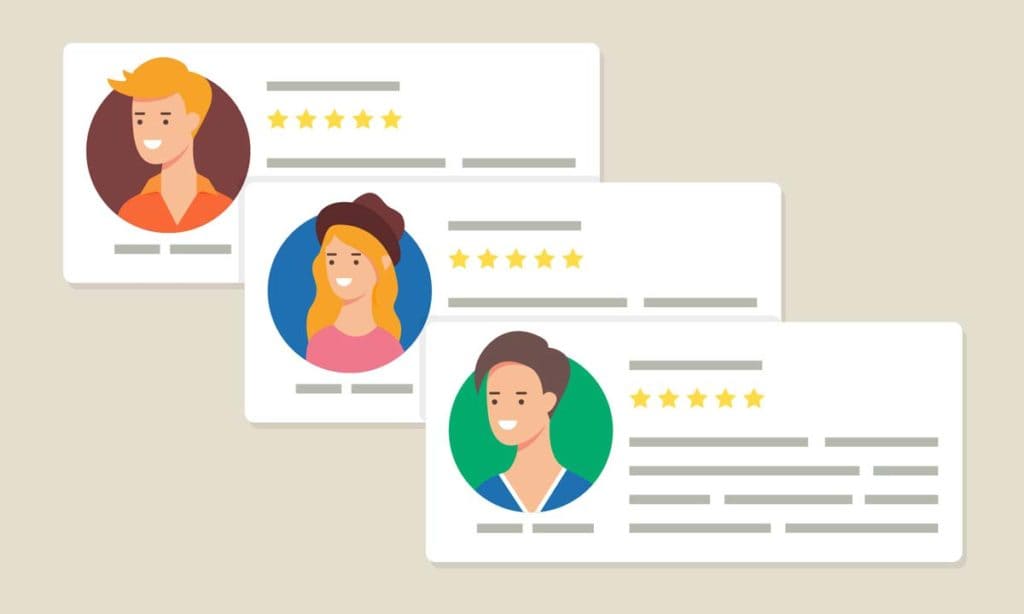
Sentiment analysis remains an active research area with exciting innovations across various techniques. Here, we explore a few promising frontiers.
Aspect-Based Sentiment Analysis
Aspect-based sentiment analysis classifies sentiment towards attributes or “aspects” of entities like products, services, organisations, etc., rather than overall sentiment.
For example, consider the hotel review:
“The room was clean, but the staff seemed rude and inattentive.”
Overall polarity = negative
But for critical aspects:
- Room cleanliness: Positive
- Staff friendliness: negative
Aspect-level analysis provides more nuanced insight for reputation monitoring and decision-making. Unique challenges include associating sentiment with the correct entity/aspect in long reviews with many targets.
Multimodal Sentiment Analysis
Most techniques focus exclusively on text, but multimodal sentiment analysis additionally considers images, audio, and video.
This can help better understand sentiment from social media posts, vlogs, broadcasts, and other media.
For example, text comments on a YouTube video may convey positive sentiment, but negative vocal inflexions in the audio could indicate sarcasm. Fusing sentiment signals from all modalities provides a richer perspective.
Temporal Sentiment Analysis
Aggregate opinions fluctuate dynamically across time. Temporal sentiment analysis tracks how sentiment around entities evolves hourly, daily, or weekly.
For example, public sentiment towards politicians can shift quickly in response to scandals, speeches, policy changes, etc. Temporal analysis helps explain spikes and dips.
Time series forecasting models can also predict future sentiment levels based on historical patterns. This is valuable for anticipating reputation risk.
Explainable Sentiment Analysis
Many advanced machine learning models act as “black boxes”, providing no visibility into why specific sentiment predictions were made.
Explainable AI solutions for sentiment analysis shed light on model behaviour by exposing influential features, textual rationales linked to predictions, counterfactuals, and more.
For example, highlighting the excerpt “ruined the whole experience” explains why a negative classification was made on a hotel review, building appropriate trust.
Real-World Deployment Considerations
Sentiment analysis delivers tremendous value across many domains when thoughtfully productionised. Here, we review critical factors for applied deployments.
Data Imbalance Handling
Real-world sentiment data often exhibits class imbalance, where one sentiment type is far more prevalent. For example, product reviews tend to be overwhelmingly positive.
Models can become biased, always predicting the majority class. Oversampling minority classes or penalising models for misclassifying these cases helps address the imbalance.
Domain Adaptability
Language use and context vary enormously across different verticals like social media, financial news, healthcare surveys, etc. Solutions pre-trained on generic text often need to be generalised. Adaptation techniques like transfer learning enable specialisation to new domains.
Data Drift Handling
Language evolves dynamically, yet models deployed to production can decay in accuracy as data drifts. Continual learning methods identify when distributions begin to shift and trigger retraining to maintain robustness.
Cloud Scalability
Sentiment analysis workloads often involve processing high volumes of streaming text from multiple sources: serverless platforms scale dynamically, analysing sentiment across thousands of posts per second without overhead.
By considering challenges like class imbalance, domain differences, concept drift, and scale, sentiment analysis systems can deliver reliable results in applied settings.
FAQs About Sentiment Analysis
We’ll conclude this overview of sentiment analysis with answers to a few frequently asked questions for additional context:
Is sentiment analysis still an active research area?
Many open challenges remain around detecting complex linguistic phenomena like sarcasm, handling domain differences, and adapting to informal language on social media. So, while core techniques are established, many unsolved problems remain.
What background is required to work in sentiment analysis?
Coursework in natural language processing, machine learning, and data science serves as good preparation. Familiarity with linguistics, like parts of speech and semantics, also provides functional fundamentals. Comfort with programming languages like Python is also a prerequisite.
How accurate are modern sentiment analysis solutions?
State-of-the-art accuracy exceeds 90% on domains with well-formatted text like movie reviews or product surveys. Performance degrades on noisy social media posts and other informal text, though. There is still much progress to be made, especially on robustness.
What is the future outlook for this field?
Sentiment analysis will continue expanding in scope and importance as more text data is generated across sectors. Understanding stakeholder perceptions through opinions shared online enables better decision-making and significant business value, further accelerating adoption.
Are there any limitations or dangers with applying sentiment analysis irresponsibly?
While there is tremendous upside, deployments could perpetuate issues like bias if the assumptions and uncertainties behind a model are not well understood. Practitioners must be ethical, transparent, and aware of limitations.
Hopefully this overview has enhanced your understanding of modern sentiment analysis. Please reach out with any other questions!
Last update on 2025-07-08 / Affiliate links / Images from Amazon Product Advertising API